Responsible AI:
Urgent Lessons for Managing Enterprise AI Risk and Reward
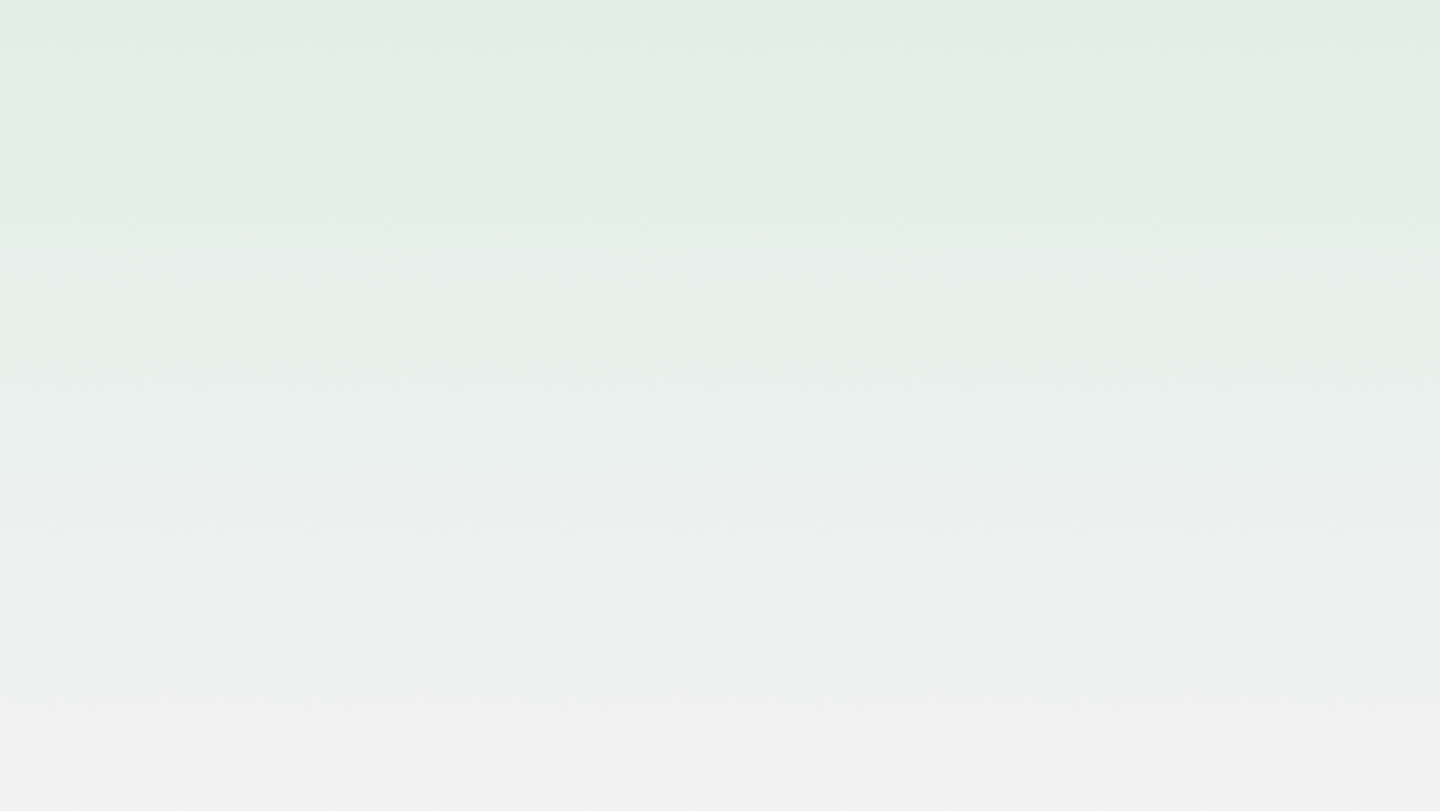
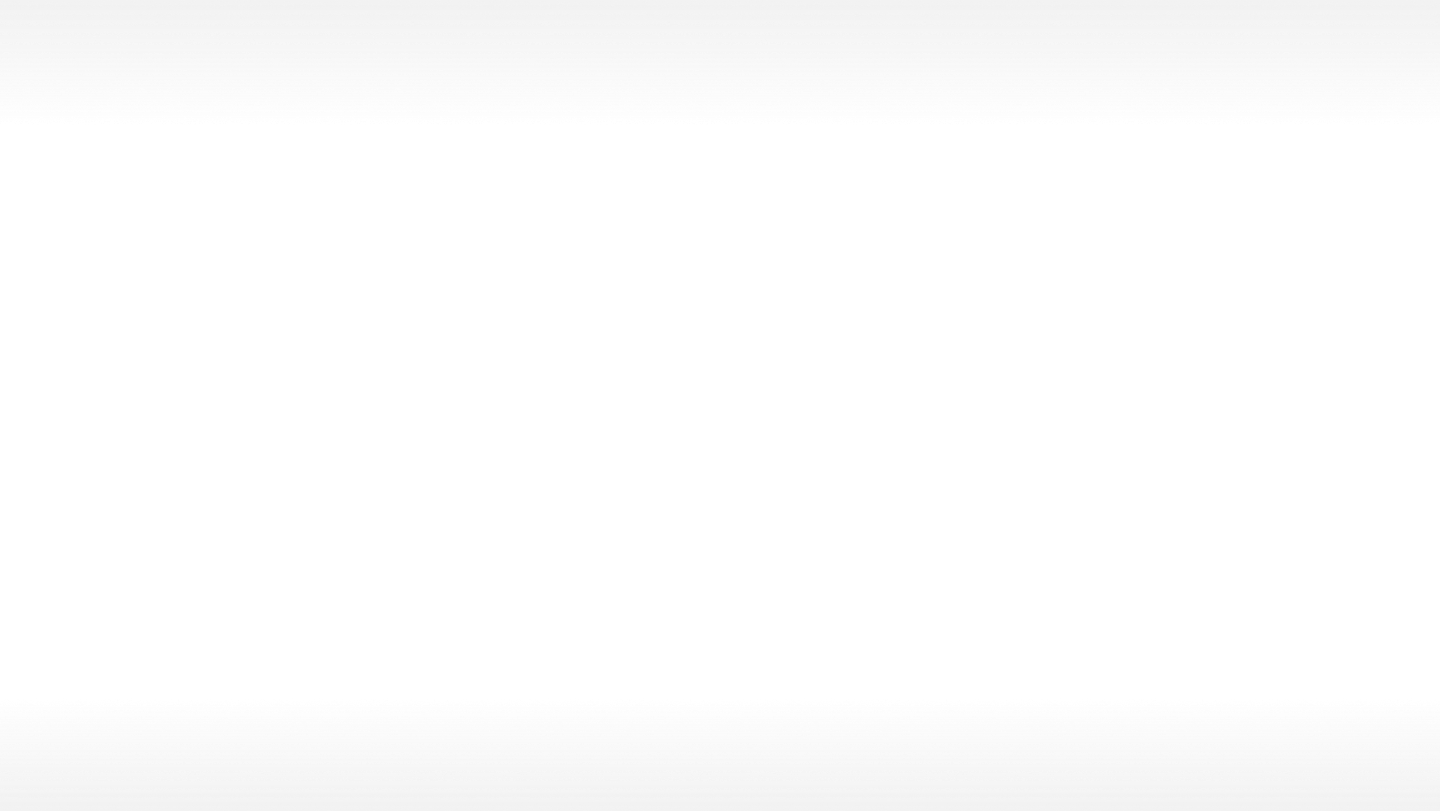
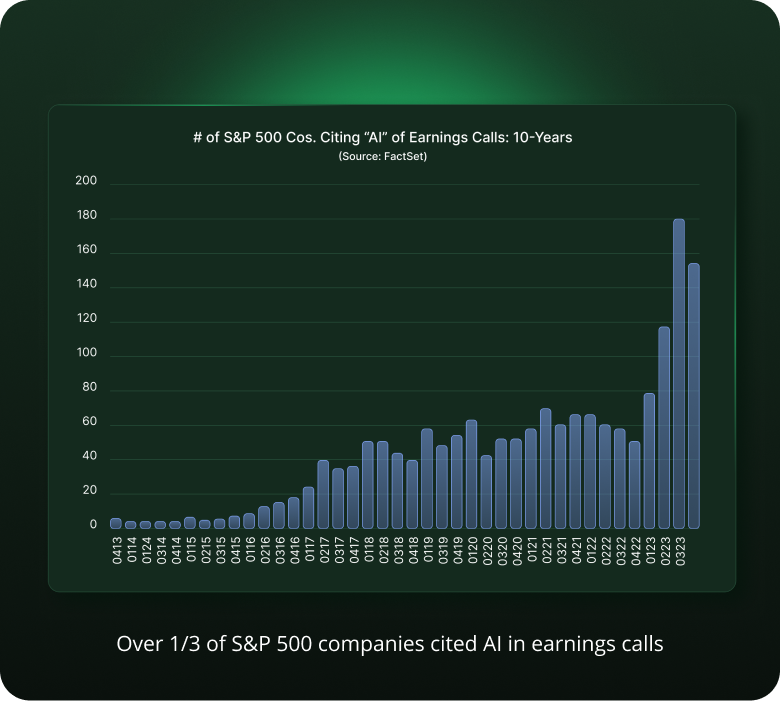
What is Enterprise AI?
Enterprise AI is the combination of Artificial Intelligence’s human-like learning and interaction abilities with custom-designed software catering to an enterprise's needs. This union can transform operations and decision-making within a business. When done effectively, the integration of AI into an organization's technology stack improves processes, intelligence, and capabilities in a safe, ethical, and responsible way. As companies learn to harness this transformative technology, they can drive innovation, productivity, and competitiveness on an enterprise scale.
Unlocking the transformational value of Enterprise AI requires effective AI Governance that delivers on business demands while safeguarding the organization from the technology's inherent risks without stifling innovation.
Why AI is Existentially Important to the Enterprise
While modern AI – especially Generative AI – is still in its infancy, it has the potential to be a transformative technology, impacting society much like the Industrial Revolution or the advent of the Internet. A quick Google search for “AI Revolution” returns dozens of books, such as the highly respected The AI Revolution in Medicine. The Founder and Executive Chairman of the World Economic Forum, Klaus Schwab, writes about this concept in depth in his book and numerous articles on The Fourth Industrial Revolution.
Cloud Computing, Big Data, and AI work together to produce capabilities on an almost inconceivable scale. Unlimited computing power, and diverse data sets — both structured and unstructured — fuel comprehensive business intelligence and analytics. AI enables companies to leverage these vast data stores and take action on the overflowing amounts of information, driving operational improvements and unlocking new insights and opportunities.
Cloud Computing
Cloud computing environments play a pivotal role in supporting AI applications by furnishing the requisite computational power for processing and organizing data in a scalable and adaptable architecture.
Furthermore, the cloud facilitates broader accessibility for enterprise users, thereby democratizing AI capabilities and expanding their reach.
Big Data
AI, in tandem, depends on large volumes of data for training and deriving valuable insights.
The capacity of AI to deliver accurate predictions, distinct from replicating human biases, necessitates both a substantial quantity and high quality of data.
AI, ML, and Deep Learning
Deep learning is a computational approach that analyzes extensive datasets to uncover nuanced patterns and correlations, offering businesses a strategic advantage.
What Are Enterprise AI Use Cases?
An AI use case is a specific business challenge or opportunity that AI may solve. By comparison, AI systems, initiatives, applications, and models are software programs grounded in Artificial Intelligence that showcase practical utility within distinct tasks, purposes, or industries. These applications leverage AI algorithms and Machine Learning techniques to address specific needs or challenges in various sectors such as healthcare, investment management, banking, insurance, CPG, retail, and government.
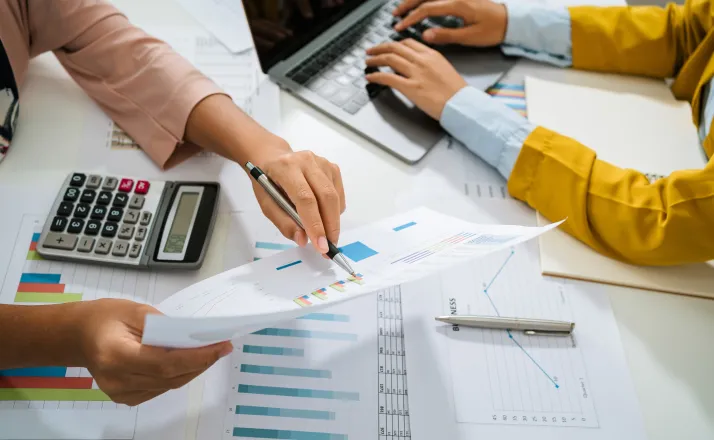
Financial Services
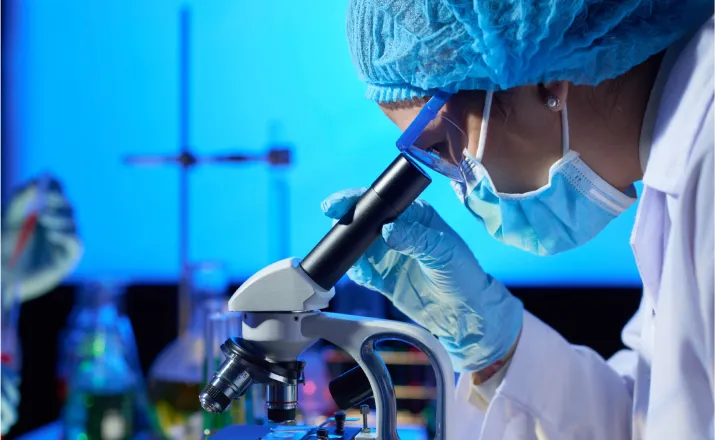
Healthcare, Pharmaceuticals, & Biotech

Consumer Packaged Goods & Retail
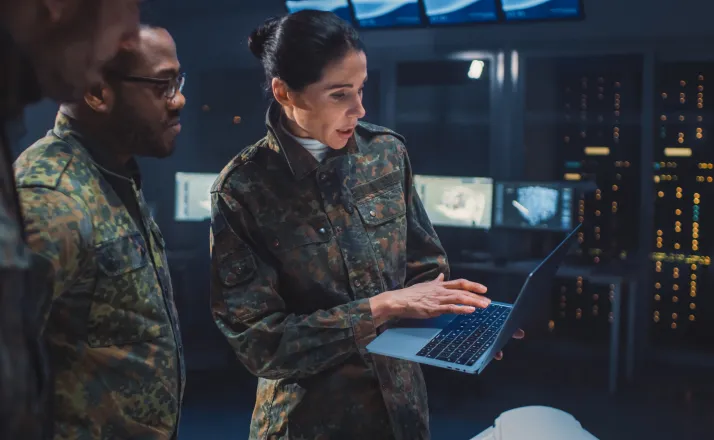
Defense, Government, & Public Sector
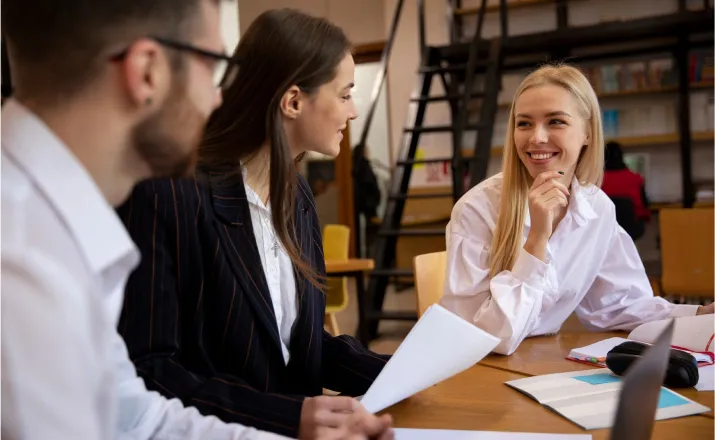
Human Resources & Payroll
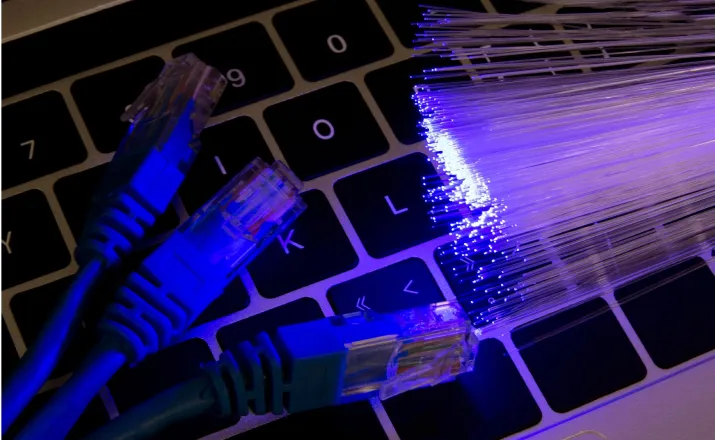
Telecommunications
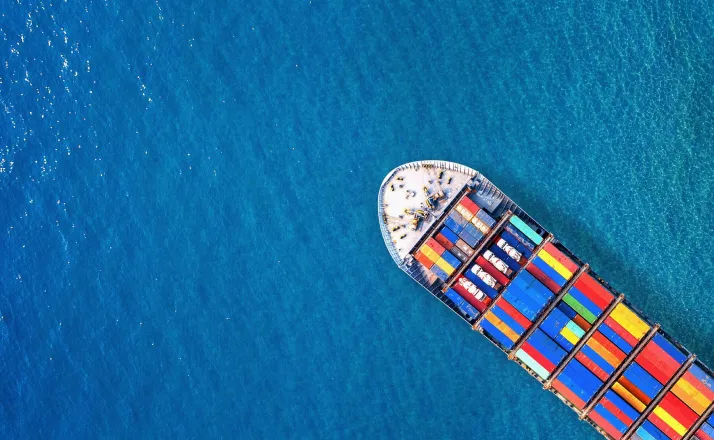
Logistics
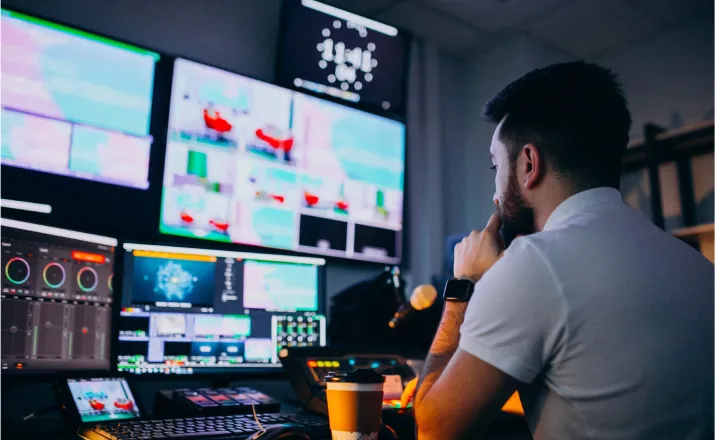
Media & Entertainment
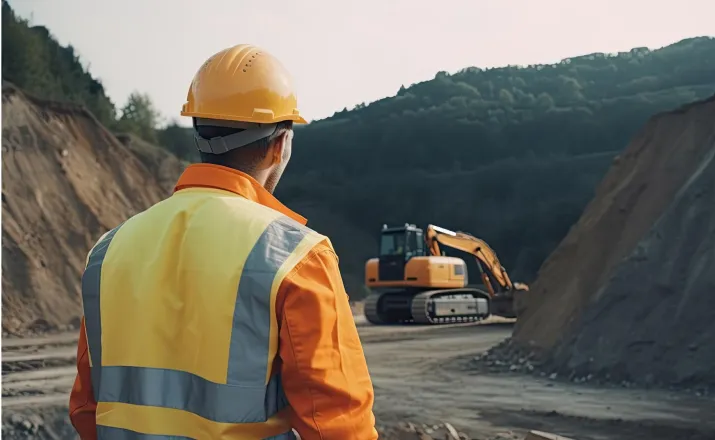
Energy, Mining, & Utilities
Governing AI is Fundamentally Different Than Governing Traditional Software and Data
Enterprise AI requires a new approach to governance because AI can be non-deterministic. In the past, development teams produced deterministic software and worked with operations and governance team in a static, one-time manner because once an initiative was approved, the outputs of the software stayed the same. This worked fine in the old days of classic software and regression models, but governance because a dirty word because it became a slow process and a heavy lift. However, generative AI changed the world, because its outputs are non-deterministic, which requires a new approach for how development, operations, and governance teams work together: Continuous AI Governance.
In the era of generative AI, all teams need to move fast and continually review AI outputs, because the outputs of AI can change instantly - within a day, hour, or even minute. Governance needs to be fast, efficient, and continuous to keep up with the demands of the business and the inherent risks that the technology presents. This is a seismic shift in the governance landscape, and as a result, enterprises require continuous AI Governance.
The Old Way: Static, Siloed Governance
Governance processes are slow, siloed, and designed for traditional software development and data management. The goal is primarily risk mitigation, and fairly or not, it's why governance may be perceived as the "department of no."
The New Way: Continuous Governance
Governance processes are dynamic, enterprise-wide, and real-time to handle changing data, inputs, and outputs. The goal is to accelerate innovation while also safeguarding the enterprise, making governance a business partner.
What is AI Governance?
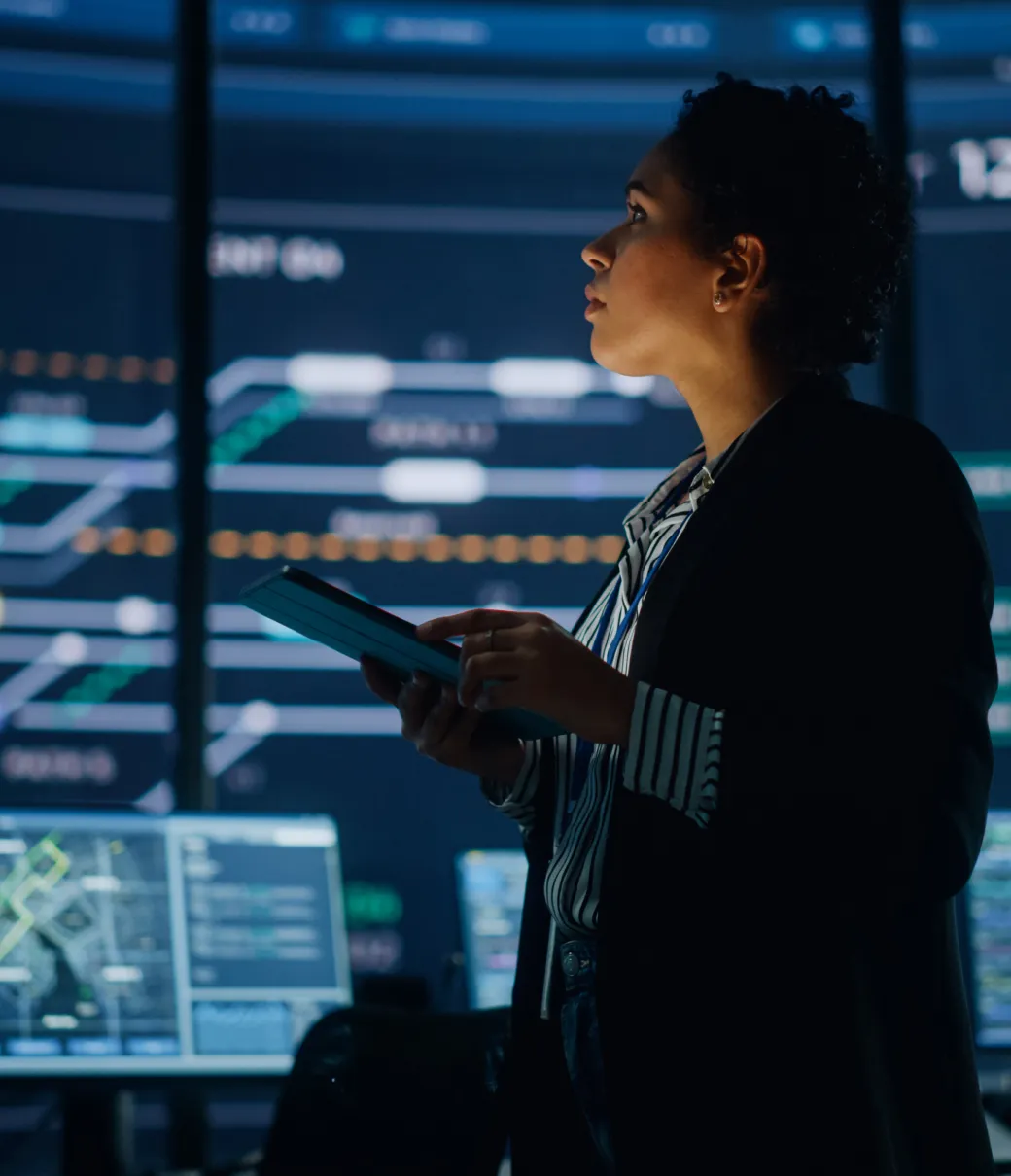
Minimum Viable Governance (MVG):
How to Quickly and Confidently Protect the Enterprise from AI Risks
.webp)
Governance Inventory
Establish visibility into all your AI initiatives with a dynamic inventory that integrates with your priority AI systems
.webp)
Light Controls
Implement a risk-based compliance approach and enforce the requisite controls for all AI systems
.webp)
Reporting
Report on AI usage, risks, and adherence across internally developed, proprietary, vendor, and embedded AI
Unlock the Transformational Value of Enterprise AI
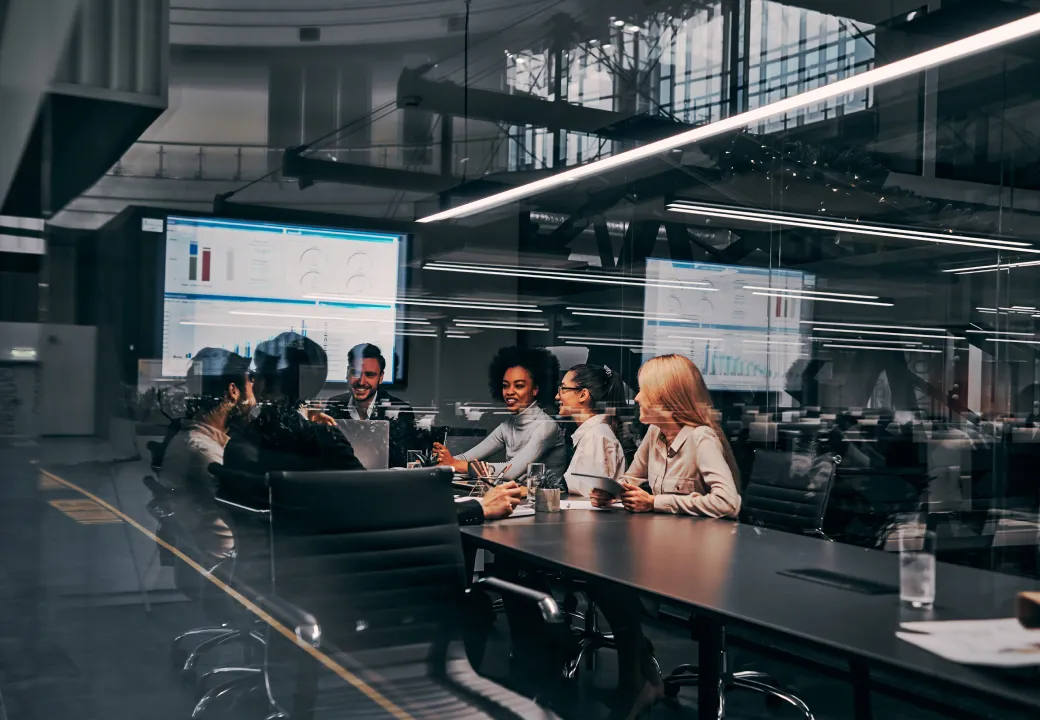
Protect the Business
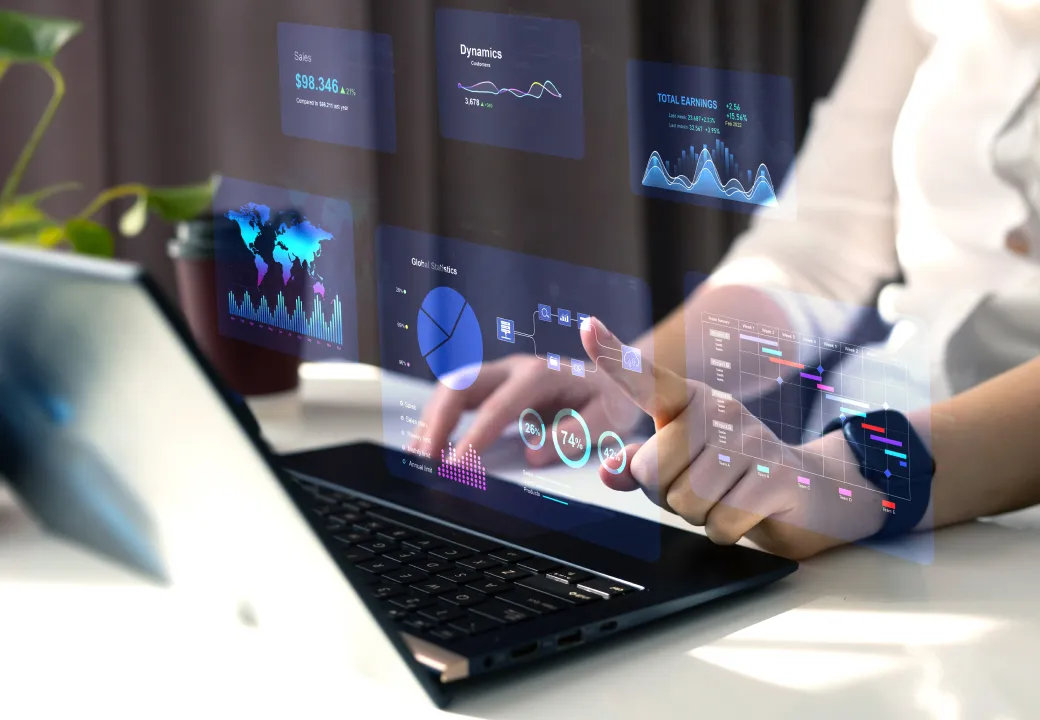
Visibility and Efficient Management
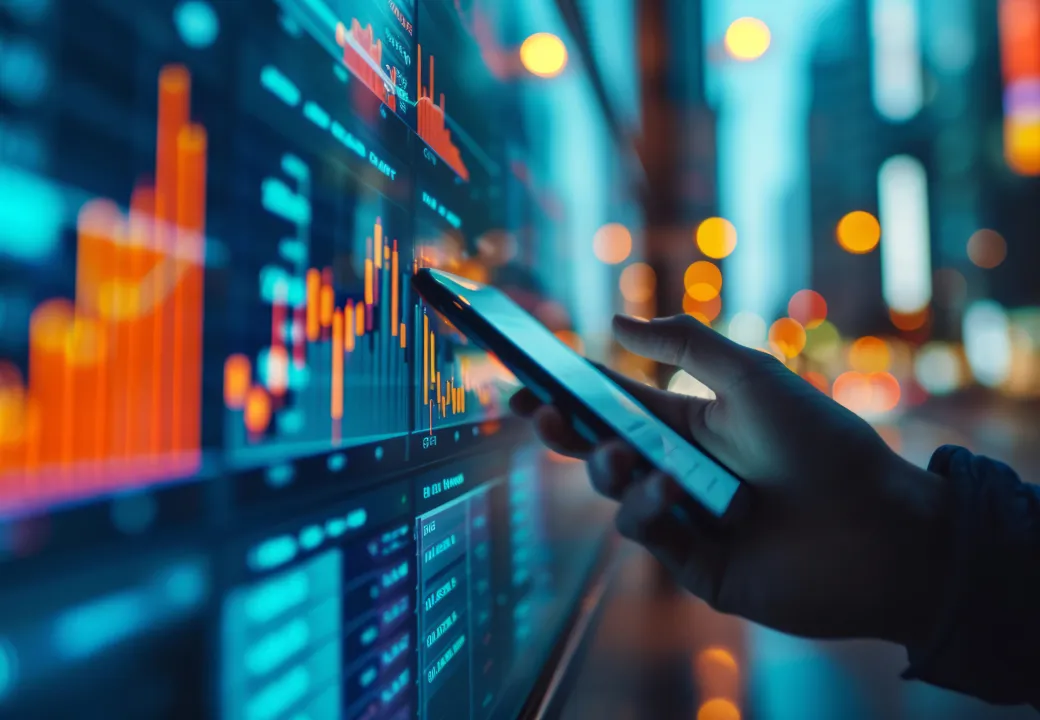
Scale and Innovation
Generative AI Necessitates AI Governance Now
The exciting potential of Generative AI for enterprises lies in the power of business transformation. By harnessing the capabilities of Generative AI within enterprise AI Governance frameworks, organizations can achieve unparalleled efficiency, elevate customer service experiences, and unlock new possibilities to propel success and build competitive advantage in a rapidly evolving technological and regulatory landscape.
Top Generative AI Use Cases for the Enterprise
Chat-Based Interfaces Embedded in Products
Coding Assistants
Customer Communications & Contact Centers
Knowledge Assistants (e.g. sales copilot)
IT Automation & Cybersecurity
Content Development (e.g. marketing personalization)
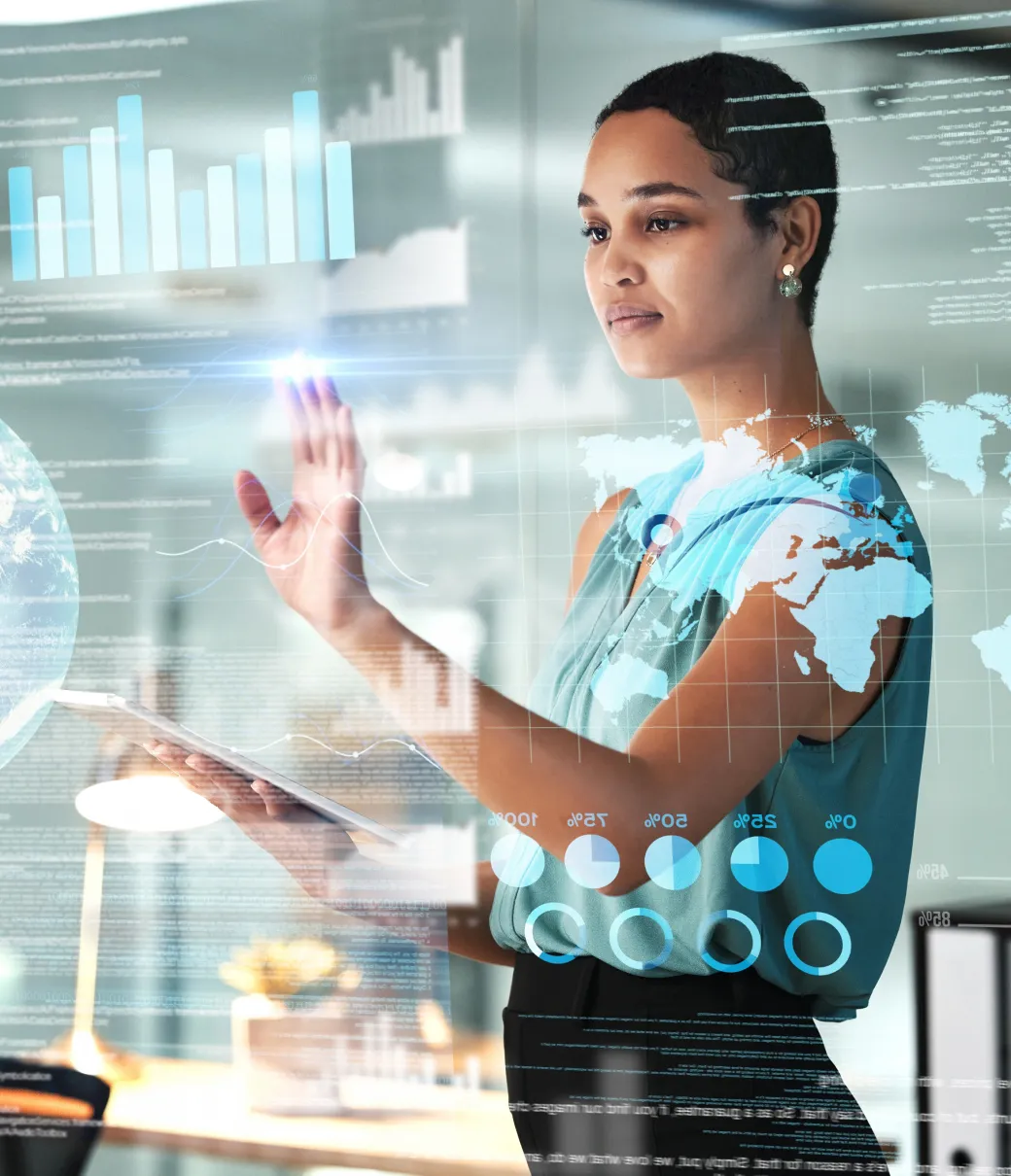
AI Regulations are Evolving Rapidly
In the evolving landscape of AI regulations, enterprise attention to compliance is imperative. Governments and regulatory bodies are increasingly scrutinizing AI practices, particularly in the financial sector. To navigate this complex terrain, enterprises must ensure their AI initiatives consider emerging regulations. Embracing AI Governance becomes not just an operational choice but a strategic imperative, safeguarding businesses from legal risks and fostering responsible innovation in line with evolving regulatory standards.
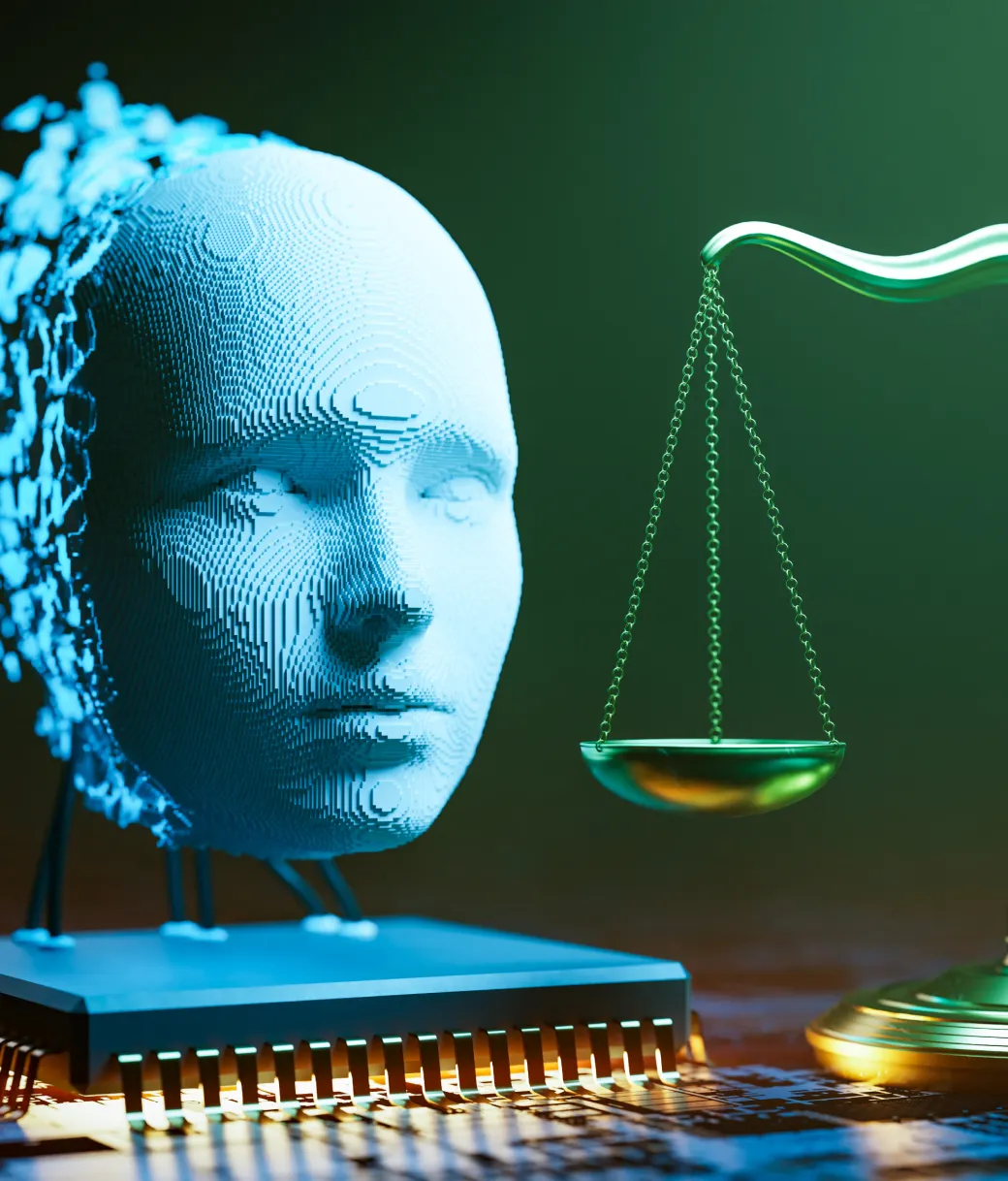
AI Governance is a Fundamental Requirement for Responsible AI
Implementing AI in the enterprise necessitates a strategic approach encompassing comprehensive AI Governance. Initiate by defining clear objectives and assessing data readiness. Establish robust governance frameworks to ensure ethical use, regulatory compliance, and risk mitigation. Integration of responsible AI practices safeguards innovation, fostering a secure and ethical AI ecosystem within the enterprise.
.webp)
Govern and Scale All Your Enterprise AI Initiatives
Discuss your AI and Governance needs with our experts